논문 링크: https://arxiv.org/pdf/2012.14740.pdf
Github: https://github.com/microsoft/unilm
GitHub - microsoft/unilm: Large-scale Self-supervised Pre-training Across Tasks, Languages, and Modalities
Large-scale Self-supervised Pre-training Across Tasks, Languages, and Modalities - GitHub - microsoft/unilm: Large-scale Self-supervised Pre-training Across Tasks, Languages, and Modalities
github.com
hugging face: https://huggingface.co/docs/transformers/model_doc/layoutlmv2
LayoutLMV2
Although the recipe for forward pass needs to be defined within this function, one should call the Module instance afterwards instead of this since the former takes care of running the pre and post processing steps while the latter silently ignores them.
huggingface.co
Introduction
- information extraction task에서 text 정보뿐만 아니라, visual과 layout 정보를 이용하는 것이 중요
- 문서의 타입이 매우 다양하기 때문에 정확한 인식을 위해 text, visual, layout 정보들이 잘 결합될 수 있도록 jointly cross-modality 모델을 설계하는 것이 필요함
- 기존의 연구들은…
- pre-train된 NLP 모델과 CV모델 사용
- 문서의 type이 바뀌면 재학습 해야 하는 단점이 존재
- 따라서, 본 논문에서는!
- 다양한 타입의 문서에 한번에 학습을 진행해 아주 적은 수의 데이터로도 충분히 fine-tuning 될 수 있도록 함
- document text, layout, visual 정보를 통합하기 위한 pre-training stage를 포함하는 multi-modal Transformer model 제안 ⇒ 하나의 framework 안에서 modal들 사이의 상호작용을 학습할 수 있도록 함
Architecture
LayoutLMv2
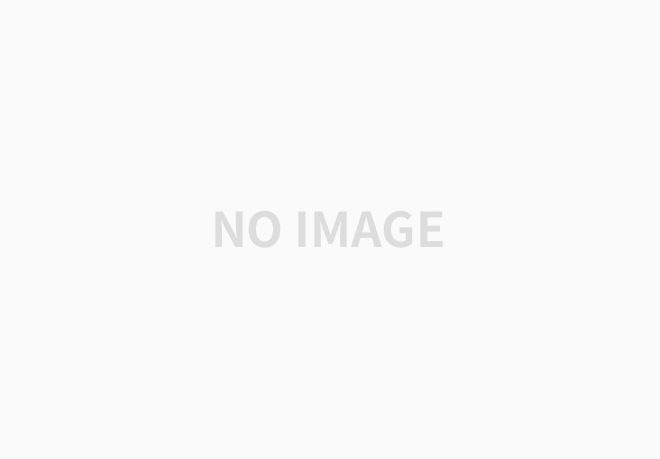
- Text Embedding
- Tokenizer:
- WordPiece
- [CLS] token: sequence의 시작 부분에 추가
- [SEP] token: sequence의 마지막 부분에 추가
- [PAD] token: sequence의 길이를 최대 길이에 맞추기 위해 나머지 부분에 추가
- 최종 text embedding
- token embedding + 1D positional embedding(token의 id embedding) + segment embedding(token의 x, y 좌표)
- Tokenizer:
- Visual Embedding
- image visual token embedding + 1D positional embedding(token의 id embedding) + segment embedding(visual token)
- Layout Embedding
- token의 x, y, w, h embedding
- Multi-modal Encoder with Spatial-Aware Self- Attention Mechanism
- 초기 input
- text embedding + visual embedding + layout embedding
- 12개의 multi-head self-attention layers
- 기존의 self attention과 다르게 다양한 spatial 정보 이용 ⇒ 여러 bbox들 사이의 다양한 relative relationship을 학습할 수 있도록 함
- attention score(value)
- hidden states
- 초기 input
Pre-training Tasks
- Masked Visual Language Modeling
- Text-Image Alignment
- Text-Image Matching
Experiment
Relation Extraction Task
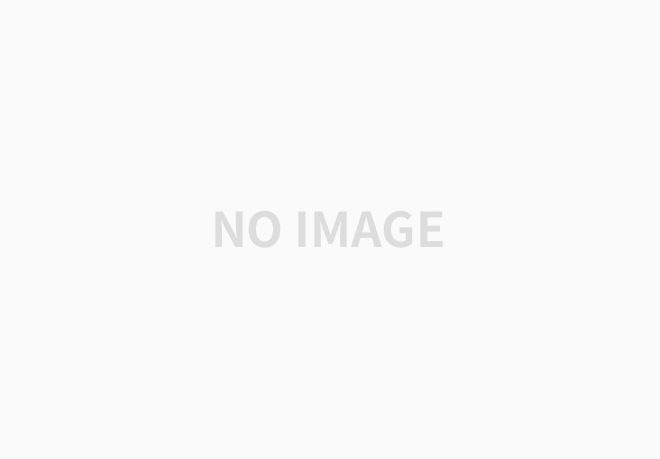
⇒ Transformer를 사용하는 다른 모델들이나 LayoutLM모델 시리즈들과 비교하여 높은 성능을 내고 있음을 알 수 있다.
Conclusion
- test, layout, image 정보를 하나의 multi-modal framework에 pre-training 시킴
- 기존의 self attention과 다르게 다양한 spatial 정보를 이용하여 여러 bbox들 사이의 다양한 relative relationship을 학습할 수 있도록 함