논문 링크 : https://onlinelibrary.wiley.com/doi/full/10.4218/etrij.2019-0093
<Introduction>
이전 논문과 비슷하게 관계 탐지에 있어 자연어를 사용하고자 한 논문입니다.
▶ ‘관계’를 language, visual, spatial cue로 연관지어 생각
- language: monkey와 banana는 ‘wear’보다 ‘eat’이 더 적합함
- visual: 이미지 feature
- spatial: banana는 monkey의 손과 입에 더 가까이 있을 수 있음
=> 세 가지 정보가 서로 다른 네트워크를 거치도록 설계
▶ 보다 정교한 언어 공간벡터를 얻기 위해 노력
<Model>
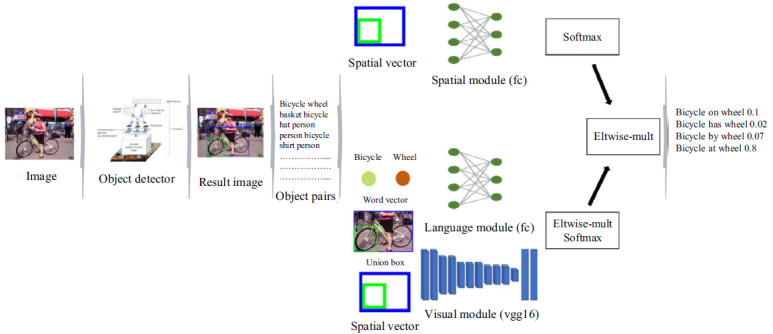
1. Object Detection
- Faster R-CNN사용
2. 다양한 모듈들
▶ Spatial Module
주어 물체와 목적어 물체의 위치 정보
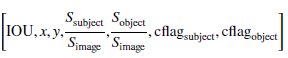
▶Langauge Module
물체 예측 클래스의 단어벡터 사용
L loss와 K loss:
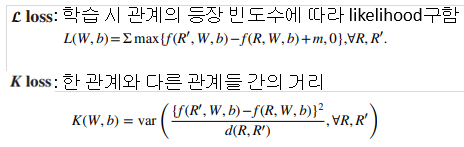

비슷한 종류의 단어들은 vector space에서 비슷한 위치에 놓이게 됨
▶Visual Module
VGG-16을 이용하여 추출
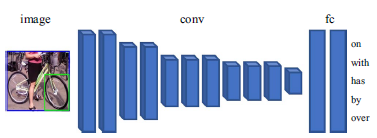
3. Relationship Detection
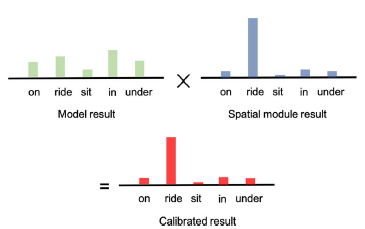
spatial module의 결과와 language+visual module의 결과를 합하여 최종 관계 클래스 결정
<Result>
Dataset
- Visual Genome
- VRD
Metrics
- Predicate detection: relation만 예측
- Phrase detection: object class와 relation예측
- Relationship detection: 물체의 영역, class, relation 모두 예측
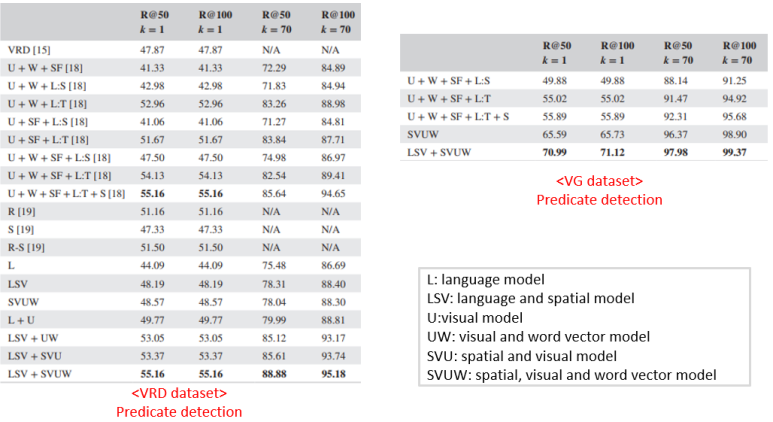
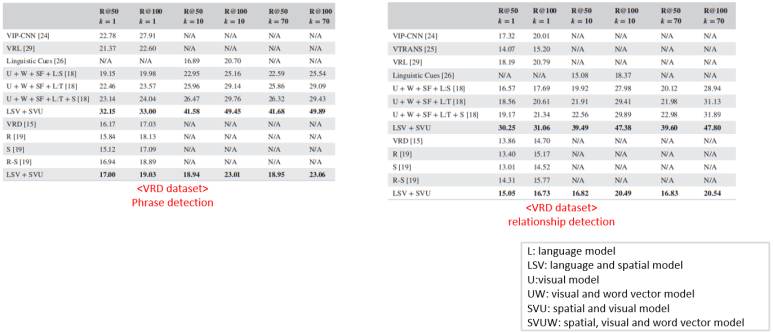
<결론>
- language, visual, spatial 정보를 모두 사용하는 관계 탐지 모델 제안
- 비슷한 종류의 물체는 비슷한 vector space에 위치 시키기 위한 language module 제안